How scientists trained computers to forecast COVID-19 outbreaks weeks ahead
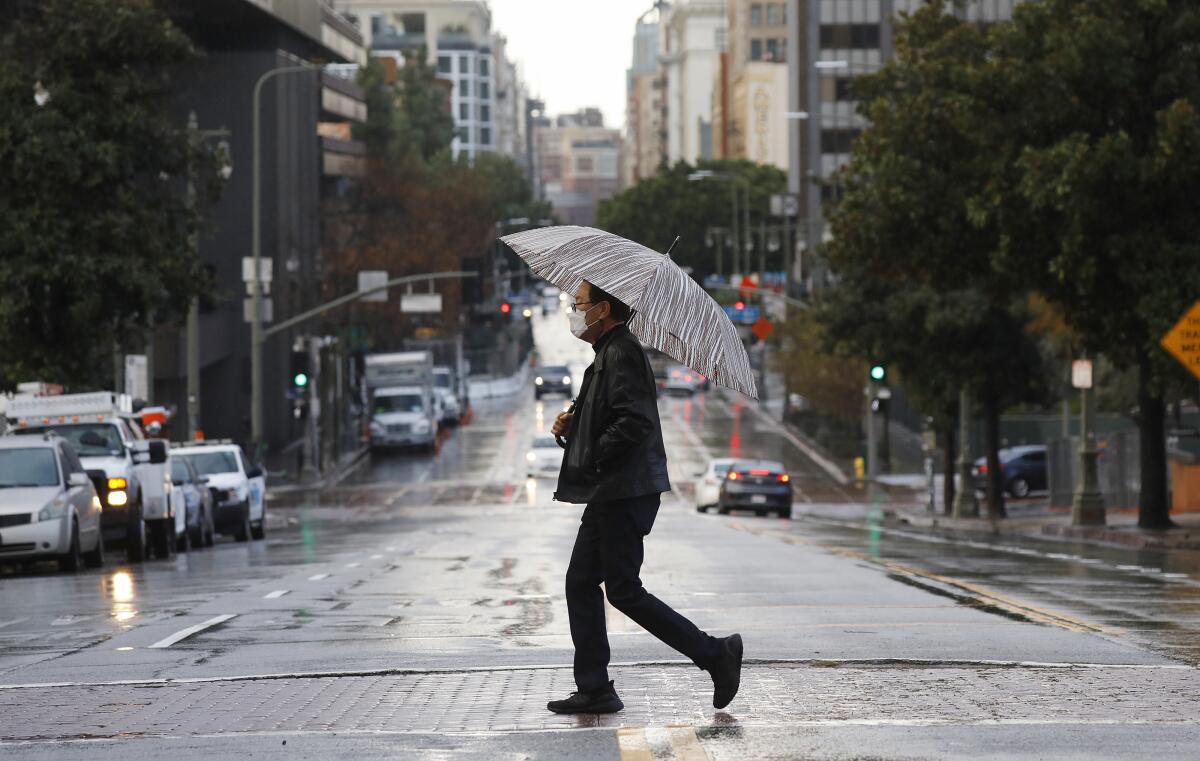
- Share via
Imagine a time when your virus-blocking face-covering is like an umbrella. Most days, it stays in your closet or is stowed somewhere in your car. But when a COVID-19 outbreak is in the forecast, you can put it to use.
Beyond that, an inclement viral forecast might induce you to choose an outdoor table when meeting a friend for coffee. If catching the coronavirus is likely to make you seriously ill, you might opt to work from home or attend church services online until the threat has passed.
Such a future assumes that Americans will heed public health warnings about the pandemic virus — and that is a big if. It also assumes the existence of a system that can reliably predict imminent outbreaks with few false alarms, and with enough timeliness and geographic precision that the public will trust its forecasts.
A group of would-be forecasters says it’s got the makings for such a system. Its proposal for building a viral weather report was published this week in the journal Science Advances.
Like the meteorological models that drive weather forecasts, the system to predict COVID-19 outbreaks emerges from a river of data fed by hundreds of streams of local and global information. They include time-stamped internet searches for symptoms such as chest tightness, loss of smell or exhaustion; geolocated tweets that include terms like “corona,” “pandemic,” or “panic buying”; aggregated location data from smartphones that reveal how much people are traveling; and a decline in online requests for directions, indicating that fewer folks are going out.
The resulting volume of information is far too much for humans to manage, let alone interpret. But with the help of powerful computers and software trained to winnow, interpret and learn from the data, a map begins to emerge.
If you check that map against historical data — in this case, two years of pandemic experience in 93 counties — and update it accordingly, you may have the makings of a forecasting system for disease outbreaks.
When everyone around them stops taking pandemic precautions, it gets harder for immunocompromised Americans to protect themselves against COVID.
That’s exactly what the team led by a Northeastern University computer scientist has done. In their bid to create an early-warning system for COVID-19 outbreaks, the study authors built a “machine learning” system capable of chewing through millions of digital traces, incorporating new local developments, refining its focus on accurate signals of illness, and generating timely notices of impending local surges of COVID-19.
Among the many internet searches it scoured, one proved to be a particularly good warning sign of an impending outbreak: “How long does COVID last?”
When tested against real-world data, the researchers’ machine-learning method anticipated upticks of local viral spread as many as six weeks in advance. Its alarm bells would go off roughly at the point where each infected person was likely to spread the virus to at least one more person.
Put to the test of anticipating 367 actual countywide outbreaks, the program provided accurate early warnings of 337 of them, or 92%. Of the remaining 30 outbreaks, it recognized 23 just as they would have become evident to human health officials.
Once the Omicron variant began to widely circulate in the U.S., the early-warning system was able to detect early evidence of 87% of outbreaks at the county level.
The distressing development comes even as other metrics show a relatively promising picture, including a decrease in case counts.
A predictive system with these capabilities might prove useful for local, state and national public health officials who need to plan for COVID-19 outbreaks and warn vulnerable citizens that the coronavirus is threatening an imminent local resurgence.
But “we’re looking beyond” COVID, said Mauricio Santillana, who directs Northeastern’s Machine Intelligence Group for the Betterment of Health and the Environment.
“Our work is aimed at documenting what techniques and approaches might be useful not just for this, but for the next pandemic,” he said. “We’re gaining trust from public health officials so they won’t need more convincing” when another disease begins spreading across the country.
That may not be an easy sell to state public health agencies and the U.S. Centers for Disease Control and Prevention, all of which struggled to keep up with pandemic data and incorporate new methods of tracking the virus’ spread. The CDC’s inability to adapt and communicate effectively during the pandemic led to some “pretty dramatic, pretty public mistakes,” Dr. Rochelle Walensky, the agency’s director, has acknowledged. Only “changing culture” will prepare the agency for the next pandemic, she warned.
Infectious disease control may be in CDC’s DNA, but the agency’s capabilities have not evolved to keep up with the faster speed and higher stakes of germs in the modern world.
The CDC’s lackluster efforts to develop prediction tools have not paved the way to easy acceptance, either. A 2022 assessment of forecasting efforts used by the CDC concluded that most “have failed to reliably predict rapid changes” in COVID-19 cases and hospitalizations. The authors of that assessment warned that the systems developed to date “should not be relied upon for decisions about the possibility or timing of rapid changes in trends.”
Anasse Bari, an expert in machine learning at New York University, called the new early-warning system “very promising.”
“The machine learning methods presented in the paper are good, mature and very well studied,” said Bari, who was not involved in the research. But he cautioned that in a once-in-a-lifetime emergency such as the pandemic, it would be risky to rely heavily on a new model to predict events.
For starters, Bari noted, this coronavirus’ first encounter with humankind has not produced the long historical record needed to fully test the model’s accuracy. And the pandemic’s three-year span has provided little time for researchers to recognize the “noise” that comes when so much data are thrown into a pot.
The CDC and state health departments have only begun to use epidemiological techniques such as phylodynamic genetic sequencing and wastewater surveillance to monitor the spread of the coronavirus. Using machine learning to forecast the location of coming viral surges may take another leap of imagination by these agencies, Santillana said.
The Biden administration is boosting efforts to identify and track coronavirus variants to help scientists see where the pandemic is heading next.
Indeed, accepting early-warning tools such as the one developed by Santillana’s group could require some leaps of faith too. As computer programs digest vast troves of data and begin to discern patterns that could be revealing, they often generate surprising “features” — variables or search terms that help foretell a significant event, such as a viral surge.
Even if these apparent signposts prove to accurately predict such an event, their relevance to a public health emergency may not be immediately clear. A surprising signal may be the first sign of some new trend — a previously unseen symptom caused by a new variant, for instance. But it also might seem so random to public health officials that it casts doubt on a program’s ability to predict an impending outbreak.
Santillana, who also teaches at Harvard’s School of Public Health, said that reviewers of his group’s early work responded with some skepticism to a few of the signals that emerged as warning signs of a coming outbreak. One of them — tweets referring to “panic buying” — seemed like an errant signal from machines that had latched on to a random event and infused it with meaning, he said.
He defended the inclusion of the “panic buying” signal as a revealing sign of an impending local outbreak. (After all, the initial days of the pandemic were marked by shortages of staple items including rice and toilet paper.) But he acknowledged that an early-warning system that is too “black-boxy” could meet with resistance from the public health officials who need to trust its predictions.
“I think the fears of decision-makers is a legitimate concern,” Santillana said. “When we find a signal, it’s got to be a reliable one.”